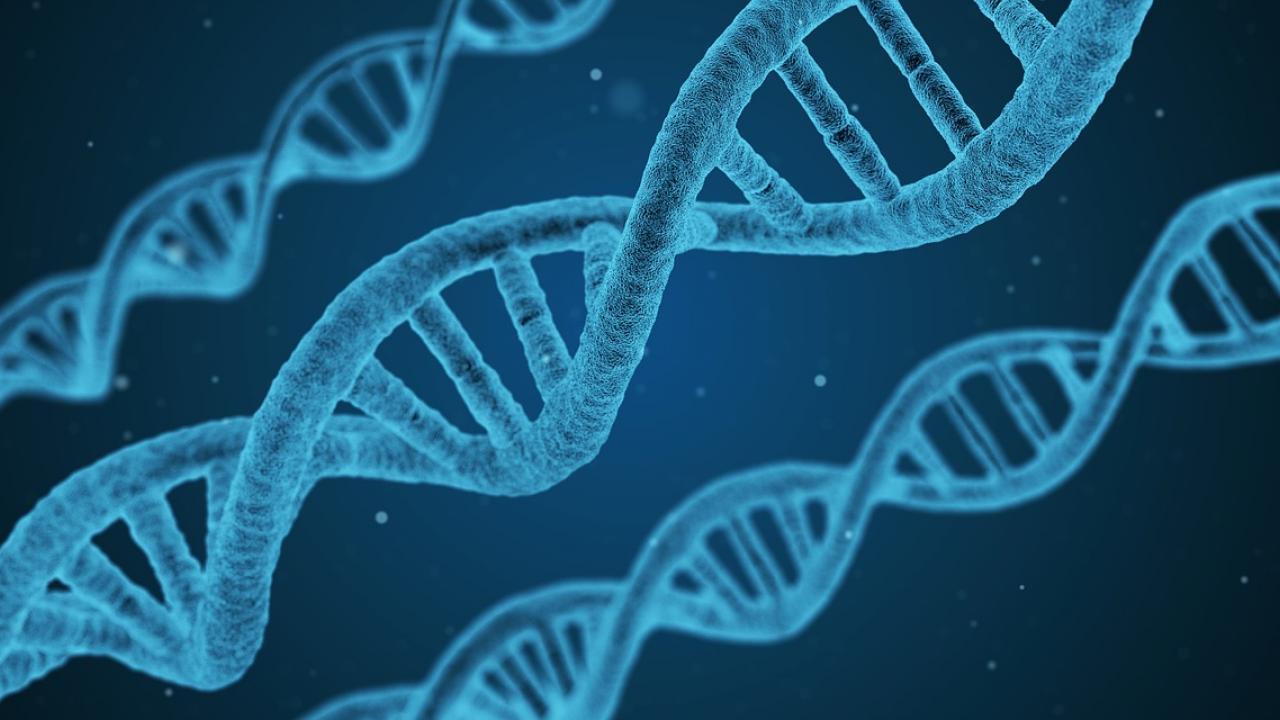
What is the Connection Between Genotype and Phenotype?
Study Describes New Statistical Method to Identify Active and Inactive Genes from Transcriptomes
Each cell of your body contains the genetic information to develop its myriad parts. From the same genetic code in each cell, development gives rise to all of the diverse tissues and organs that comprise our bodies.
Biologists have long sought to understand the relationship between genotype (the underlying genetic code) and phenotype (the resulting organismal structure).
“How is it that the cells of a multicellular organism—each with an identical genome—give rise to tissues and organs of astonishing structural and functional diversity?” asked a group of UC Davis researchers in a study appearing in Proceedings of the National Academy of Sciences.
The research team included Postdoctoral Scholar Ammon Thompson, Professor of Evolution and Ecology Artyom Kopp, Associate Professor of Evolution and Ecology Brian Moore and Population Biology Graduate Group student Michael May.
Central to answering these fundamental questions is the transcriptome: the subset of genes that are expressed in a given tissue.
Animal and plant genomes contain tens of thousands of genes, and a sizeable subset of those genes is actively expressed in each tissue. Exploring the genotype-phenotype connection requires that biologists are able to reliably identify which genes are actively expressed in which tissue. This seemingly simple task is, in fact, notoriously difficult due to both the molecular mechanics of transcription, and also to vagaries of the techniques used to collect transcriptomic data.
Like all biological processes, gene transcription is noisy; some genes that are not functional in a given tissue are nevertheless transcribed at low levels (therefore, detecting transcripts of a given gene in a given tissue does not necessarily indicate that it is active there). The technical process of collecting transcriptomic data contributes additional noise, which may cause researchers to miss some functional genes (therefore, detecting zero transcripts of a given gene in a given tissue does not necessarily indicate that it is inactive there).
According to Thompson and colleagues, these sources of noise have prevented scientists from asking apparently simple—albeit clearly important—biological questions such as “Which genes are active in a particular organ, such as the brain? Are any of these genes expressed exclusively in this organ? Were any of these genes recently activated in human evolution? And how common are such changes in gene-expression state (activation/deactivation)?”
To answer these questions, scientists must reliably disentangle the signal of active expression from various sources of noise in transcriptomic data.
The UC Davis team developed a statistical method that—by virtue of providing a mathematical description of the relevant biological and technical processes associated with transcriptomic data—allows researchers to identify the expression state of genes. Specifically, they developed a hierarchical Bayesian model that leverages patterns of variation in gene expression both among genes and between replicate transcriptome samples to isolate the signature of active expression in RNA-sequencing datasets.
“Our method provides a data-driven approach for identifying the boundary between active transcription and background noise,” said the researchers. “It takes full advantage of experimental replication and eliminates the need for ad hoc procedures”.
The researchers implemented their statistical method in the computer program, ZigZag, providing an important new tool for biologists.
ZigZag to success
The team tested the efficacy and accuracy of their method using a variety of experiments. First, the team performed an “empirical benchmark” analysis of human-lung tissues, where the expression state of each gene had previously been determined by independent means (based on chemical markers surrounding the chromosomal locations of genes). They then used ZigZag to infer the expression states of each gene from human-lung transcriptomes, which correctly inferred the (known) expression state of more than 90% of these genes.
To demonstrate the potential of their method, the team used ZigZag to compare the transcriptomes of human, chimpanzee and macaque brains. The team inferred gene-expression states in six different brain regions—the amygdala, ventral frontal cortex, dorsal frontal cortex, superior temporal cortex, striatum, and area 1 visual cortex—to identify the set of genes that are uniquely active (or inactive) in the human brain.
Across the six brain regions the researchers discovered “nine to 20 genes that were uniquely active in humans and 16 to 23 genes that were uniquely inactive in humans, with the greatest number of unique expression states located in the striatum.” Because the striatum is involved in coordinating multiple aspects of cognition, the team noted that the discovery of “genes that are uniquely active in the human brain represent factors that may be involved in human cognitive evolution.”
Commenting on the importance of the new method, the researchers said that it "will provide a powerful means to classify the expression state of genes in any sample, while at the same time quantifying the uncertainty in this classification.”
The team said they “are optimistic that—by providing a reliable and powerful means to infer the expression state of genes—our method will greatly enhance the ability of biologists to compare transcriptomes of different species and tissues and thereby to enhance our understanding of transcriptome evolution and ultimately to reveal the relationship between genotype and phenotype.”